LMStudioで外部ツールを呼び出す方法|Tool Use対応モデルの使い方
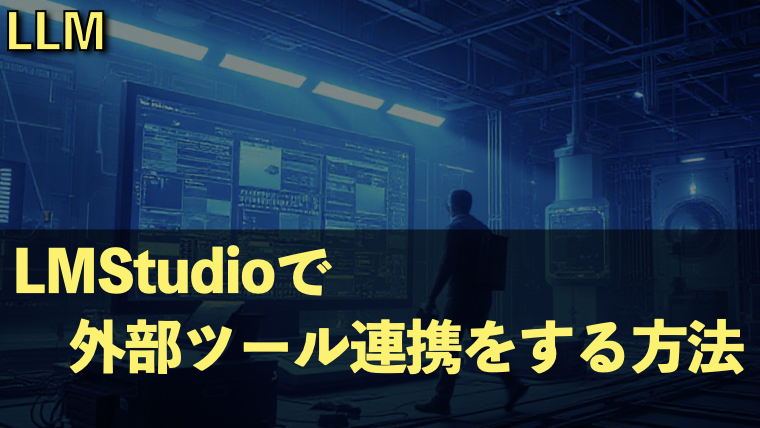
LMStudioで利用できる言語モデルの中には、工具のアイコンが表示されたものがあります。マウスを重ねると「This model has been trained for tool use.」と表示され、外部ツールとの連携機能(Tool Use)に対応していることが分かります。本記事では、LMStudioにおけるTool Useの基本的な仕組みと、実際に外部ツールを呼び出す方法を、サンプルコードと実行結果を交えて分かりやすく解説します。
LMstudioのSDK(lmstudio-python
)を利用して呼び出す方法は以下の記事を参照してください。
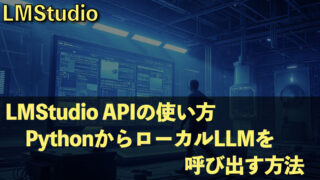
LMStudioのTool Useって?
Tool Useとは
LMStudioのTool Useは、外部ツールを使うためのインタフェースです。
Tool Useを使うことで、LLM(大規模言語モデル)が関数を呼び出すようなテキストを出力することができます。
もちろん、LLM自体は直接コードを実行することはできませんので、実際にはLLMの応答に合わせて関数を実行し、結果をLLMにフィードバックする必要があります。
これを利用すると、LLMに問い合わせ→「ツールの呼び出しを指示」→関数を実行して結果をLLMに返す→結果を使った回答を生成という流れを自動化できます。
上記の流れを見るとわかる様に、ツールは自分で定義して、呼び出す関数も用意する必要があります。このため、PythonなどのプログラムからAPI呼び出しを利用してLMStudioにアクセスする必要があります。
対応しているLLM
全てのモデルがツール利用に対応しているわけではありません。ツール利用ができるLLMは、LMStudioのモデル一覧で確認することができます。
下の図のように、ハンマー(?)のような工具のマークがあるLLMはTool Useに対応しています。

これらのモデルをAPI経由で呼び出せば、外部ツールを呼び出すことができます。
以下では、LMStudioのQuick DocsにあるTool Useを実際に試してみます。
LMstudioのSDK(lmstudio-python
)を利用して呼び出す方法は以下の記事を参照してください。
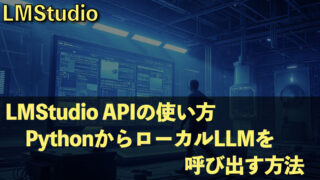
Wikipediaを参照して回答するプログラム例
Quick DocsにはToolを利用して、Wikipediaにアクセスして回答を返す例が用意されています。今回は、実際にこのコードを動かしてみました。
プログラム全体
ほぼ、quick docそのままのコードです(wikipediaを日本語リンクに変更しただけ)
"""
LM Studio Tool Use Demo: Wikipedia Querying Chatbot
Demonstrates how an LM Studio model can query Wikipedia
"""
# Standard library imports
import itertools
import json
import shutil
import sys
import threading
import time
import urllib.parse
import urllib.request
# Third-party imports
from openai import OpenAI
# Initialize LM Studio client
client = OpenAI(base_url="http://127.0.0.1:1234/v1", api_key="lm-studio")
MODEL = "qwen2.5-14b-instruct-mlx"
def fetch_wikipedia_content(search_query: str) -> dict:
"""Fetches wikipedia content for a given search_query"""
try:
# Search for most relevant article
search_url = "https://jp.wikipedia.org/w/api.php"
search_params = {
"action": "query",
"format": "json",
"list": "search",
"srsearch": search_query,
"srlimit": 1,
}
url = f"{search_url}?{urllib.parse.urlencode(search_params)}"
with urllib.request.urlopen(url) as response:
search_data = json.loads(response.read().decode())
if not search_data["query"]["search"]:
return {
"status": "error",
"message": f"No Wikipedia article found for '{search_query}'",
}
# Get the normalized title from search results
normalized_title = search_data["query"]["search"][0]["title"]
# Now fetch the actual content with the normalized title
content_params = {
"action": "query",
"format": "json",
"titles": normalized_title,
"prop": "extracts",
"exintro": "true",
"explaintext": "true",
"redirects": 1,
}
url = f"{search_url}?{urllib.parse.urlencode(content_params)}"
with urllib.request.urlopen(url) as response:
data = json.loads(response.read().decode())
pages = data["query"]["pages"]
page_id = list(pages.keys())[0]
if page_id == "-1":
return {
"status": "error",
"message": f"No Wikipedia article found for '{search_query}'",
}
content = pages[page_id]["extract"].strip()
return {
"status": "success",
"content": content,
"title": pages[page_id]["title"],
}
except Exception as e:
return {"status": "error", "message": str(e)}
# Define tool for LM Studio
WIKI_TOOL = {
"type": "function",
"function": {
"name": "fetch_wikipedia_content",
"description": (
"Search Wikipedia and fetch the introduction of the most relevant article. "
"Always use this if the user is asking for something that is likely on wikipedia. "
"If the user has a typo in their search query, correct it before searching."
),
"parameters": {
"type": "object",
"properties": {
"search_query": {
"type": "string",
"description": "Search query for finding the Wikipedia article",
},
},
"required": ["search_query"],
},
},
}
# Class for displaying the state of model processing
class Spinner:
def __init__(self, message="Processing..."):
self.spinner = itertools.cycle(["-", "/", "|", "\\"])
self.busy = False
self.delay = 0.1
self.message = message
self.thread = None
def write(self, text):
sys.stdout.write(text)
sys.stdout.flush()
def _spin(self):
while self.busy:
self.write(f"\r{self.message} {next(self.spinner)}")
time.sleep(self.delay)
self.write("\r\033[K") # Clear the line
def __enter__(self):
self.busy = True
self.thread = threading.Thread(target=self._spin)
self.thread.start()
return self
def __exit__(self, exc_type, exc_val, exc_tb):
self.busy = False
time.sleep(self.delay)
if self.thread:
self.thread.join()
self.write("\r") # Move cursor to beginning of line
def chat_loop():
"""
Main chat loop that processes user input and handles tool calls.
"""
messages = [
{
"role": "system",
"content": (
"You are an assistant that can retrieve Wikipedia articles. "
"When asked about a topic, you can retrieve Wikipedia articles "
"and cite information from them."
),
}
]
print(
"Assistant: "
"Hi! I can access Wikipedia to help answer your questions about history, "
"science, people, places, or concepts - or we can just chat about "
"anything else!"
)
print("(Type 'quit' to exit)")
while True:
user_input = input("\nYou: ").strip()
if user_input.lower() == "quit":
break
messages.append({"role": "user", "content": user_input})
try:
with Spinner("Thinking..."):
response = client.chat.completions.create(
model=MODEL,
messages=messages,
tools=[WIKI_TOOL],
)
if response.choices[0].message.tool_calls:
# Handle all tool calls
tool_calls = response.choices[0].message.tool_calls
# Add all tool calls to messages
messages.append(
{
"role": "assistant",
"tool_calls": [
{
"id": tool_call.id,
"type": tool_call.type,
"function": tool_call.function,
}
for tool_call in tool_calls
],
}
)
# Process each tool call and add results
for tool_call in tool_calls:
args = json.loads(tool_call.function.arguments)
result = fetch_wikipedia_content(args["search_query"])
# Print the Wikipedia content in a formatted way
terminal_width = shutil.get_terminal_size().columns
print("\n" + "=" * terminal_width)
if result["status"] == "success":
print(f"\nWikipedia article: {result['title']}")
print("-" * terminal_width)
print(result["content"])
else:
print(
f"\nError fetching Wikipedia content: {result['message']}"
)
print("=" * terminal_width + "\n")
messages.append(
{
"role": "tool",
"content": json.dumps(result),
"tool_call_id": tool_call.id,
}
)
# Stream the post-tool-call response
print("\nAssistant:", end=" ", flush=True)
stream_response = client.chat.completions.create(
model=MODEL, messages=messages, stream=True
)
collected_content = ""
for chunk in stream_response:
if chunk.choices[0].delta.content:
content = chunk.choices[0].delta.content
print(content, end="", flush=True)
collected_content += content
print() # New line after streaming completes
messages.append(
{
"role": "assistant",
"content": collected_content,
}
)
else:
# Handle regular response
print("\nAssistant:", response.choices[0].message.content)
messages.append(
{
"role": "assistant",
"content": response.choices[0].message.content,
}
)
except Exception as e:
print(
f"\nError chatting with the LM Studio server!\n\n"
f"Please ensure:\n"
f"1. LM Studio server is running at 127.0.0.1:1234 (hostname:port)\n"
f"2. Model '{MODEL}' is downloaded\n"
f"3. Model '{MODEL}' is loaded, or that just-in-time model loading is enabled\n\n"
f"Error details: {str(e)}\n"
"See https://lmstudio.ai/docs/basics/server for more information"
)
exit(1)
if __name__ == "__main__":
chat_loop()
実行例
実行にあたって、必要なライブラリはインストールしてください。openaiをインストールしていない場合は、pip install openai
を実行してインストールします
LMStudioを立ち上げてサーバをONにします。これでPythonプログラムからのアクセスが可能になります。
今回はqwen2.5-14b-instruct-mlx
を利用しています(サンプルがこのモデルを使っていました)。他のモデルでもTool Use
に対応しているモデルであれば同様のことが可能だと思いますが未確認です。
動作結果
ドラゴンボールの作者についてと言わせた結果が以下になります。おそらく、LLM自体も知っているとは思いますが、Wikipediaを検索し(赤い部分)、それを元に回答しています。
このように、Wikipedaを検索して、その結果を受けてアシスタントが回答するという機能が実現できました。
Assistant: Hi! I can access Wikipedia to help answer your questions about history, science, people, places, or concepts - or we can just chat about anything else!
(Type 'quit' to exit)
You: ドラゴンボールの作者は?
====================...
Wikipedia article: ドラゴンボール
--------------------...
『ドラゴンボール』(DRAGON BALL)は、鳥山明による日本の漫画。『週刊少年ジャンプ』(集英社)において、1984年51号から1995年25号まで連載された。略称は「DB」。
===================...
Assistant: ドラゴンボールの作者は、鳥山明です。彼は1984年5月から1995年2月まで週刊少年ジャンプに連載を行いました。愛称は「DB」です。
You:
以下、コードを解説していきます。
コード解説
以下、コードを解説していきますが、私もコードを読みながら確認していっただけなので、間違って解釈している部分があるかもしれませんが、その点はご容赦ください。
1. 準備:必要なライブラリのインポート
まず、プログラムに必要な様々な機能を呼び出すための「ライブラリ」を読み込んでいます。
読み込むのは、JSONデータの処理、URLの操作、時間管理、スレッド制御、openai
ライブラリです。なお、openai
ライブラリはLM Studioと通信するためのインターフェースです。
import itertools
import json
import shutil
import sys
import threading
import time
import urllib.parse
import urllib.request
from openai import OpenAI
2. LM Studioクライアントの初期化
以下の部分では、LM Studioサーバーへの接続設定を行っています。
base_url
はLM Studioサーバーのアドレス(通常はローカルホストの1234番ポート)を指定し、api_key
は認証のためのキーです(ここではダミーの “lm-studio” を使用していますが必要ないかも?)。
MODEL
変数には、利用するLM Studioモデルの名前を格納します。このモデル名は、事前にLM Studioでダウンロード・ロードしておく必要があります。
client = OpenAI(base_url="http://127.0.0.1:1234/v1", api_key="lm-studio")
MODEL = "qwen2.5-14b-instruct-mlx"
3. Wikipedia検索機能の実装 (fetch_wikipedia_content
)
fetch_wikipedia_content
関数は、与えられた検索クエリ (search_query
) を使ってWikipediaを検索し、最も関連性の高い記事の概要(冒頭部分)を取得します。
Wikipedia APIにアクセスして検索を行い、見つかった記事の内容を辞書形式で返します。エラーが発生した場合は、status: "error"
と message
(エラー内容) を含む辞書が返されます。
この関数は、Toolの呼び出しがLLMから返された場合に利用します。
def fetch_wikipedia_content(search_query: str) -> dict:
# ... (Wikipedia APIへのアクセスとデータ取得処理) ...
return { "status": "success", "content": content, "title": pages[page_id]["title"] }
4. LM Studioへのツール定義 (WIKI_TOOL
)
この部分は、LM Studioに「Wikipedia検索機能」をツールとして登録するための設定です。
WIKI_TOOL
は、ツールの種類 (type: "function"
)、名前 (name
)、説明 (description
)、そして入力パラメータ (parameters
) を定義しています。特に description
は重要で、LM Studioモデルがこのツールを使うべきかどうかを判断するために利用されます。
WIKI_TOOL = {
"type": "function",
"function": {
"name": "fetch_wikipedia_content",
"description": (
"Search Wikipedia and fetch the introduction of the most relevant article. ... "
),
"parameters": {
"type": "object",
"properties": {
"search_query": {
"type": "string",
"description": "Search query for finding the Wikipedia article",
},
},
"required": ["search_query"],
},
},
}
5. チャットループの実装 (chat_loop
)
チャットのメインループ関数です。
def chat_loop():
# ... (会話履歴の初期化) ...
while True:
user_input = input("\nYou: ").strip()
if user_input.lower() == "quit":
break
messages.append({"role": "user", "content": user_input})
try:
with Spinner("Thinking..."):
response = client.chat.completions.create(
model=MODEL,
messages=messages,
tools=[WIKI_TOOL],
)
# ... (ツール呼び出しの処理、結果の取得と会話履歴への追加) ...
except Exception as e:
# ... (エラーハンドリング) ...
処理の流れは以下のようになります。
- ユーザーからの入力を受け取る
input()
関数でユーザーの質問を受け付けます - LM Studio APIを呼び出す
ユーザーの入力と過去の会話履歴 (messages
) をLMStudioに送信し、応答を待ちます。tools=[WIKI_TOOL]
には、利用可能なツールとしてWikipedia検索機能を指定しています - ツール呼び出しの処理
LLMからの応答にツール呼び出しが含まれている場合(つまり、モデルがWikipedia検索が必要と判断した場合)、fetch_wikipedia_content
関数を呼び出してWikipediaから情報を取得します - 結果の会話履歴への追加
取得したWikipediaの情報は、会話履歴 (messages
) に追加され、再度LMStudioにメッセージを送信します。これにより、LM Studioは以前に取得した情報に基づいて応答を生成できます - ツール呼び出しが含まれていない場合
ツール呼び出しが含まれていない場合は、応答をそのまま出力します
プログラムを見るとわかるように、LLMへメッセージを送信→ツール使用の場合はツールを使用してメッセージに追加→再度LLMへメッセージ送信を行います。
6. プログラムの実行
メイン部分です。chat_loop
を呼び出すだけです。
if __name__ == "__main__":
chat_loop()
その他
肝となるのは、ツールの登録と、ツールの使用が指定された場合にツールを呼び出してその結果を返す処理でしょうか。ツールは独自のものを追加できるので、例えば今日の天気や気温などを返すと言ったことも可能です。
また、プログラムを見ると複数のツールが呼び出された場合も想定されていますので、このプログラムをベースに複数のツールを登録して使用することも可能そうです。
自分で作った関数をLLMから呼び出せるというのは面白いと思います。ChatGPTなどでは普通に行われていましたが、ローカルLLMでも可能というのは知りませんでした。
まとめ
LMStudioをサーバーモードで利用して、Tool Use
を行うプログラムを紹介しました。紹介したプログラムでは、Wikipediaを検索して、それを元にユーザの質問に答えるというものです。このプログラムのポイントはツール定義の部分です。このような感じで独自のツールを埋め込むことができます。
LMstudioのSDK(lmstudio-python
)を利用して呼び出す方法は以下の記事を参照してください。
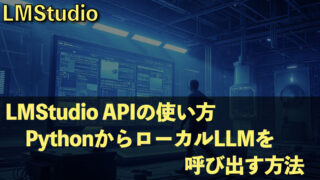
[…] LMStudioで外部ツールを呼び出す方法|Tool Use対応モデルの使い方 […]